The least talked about study design - the ecological study
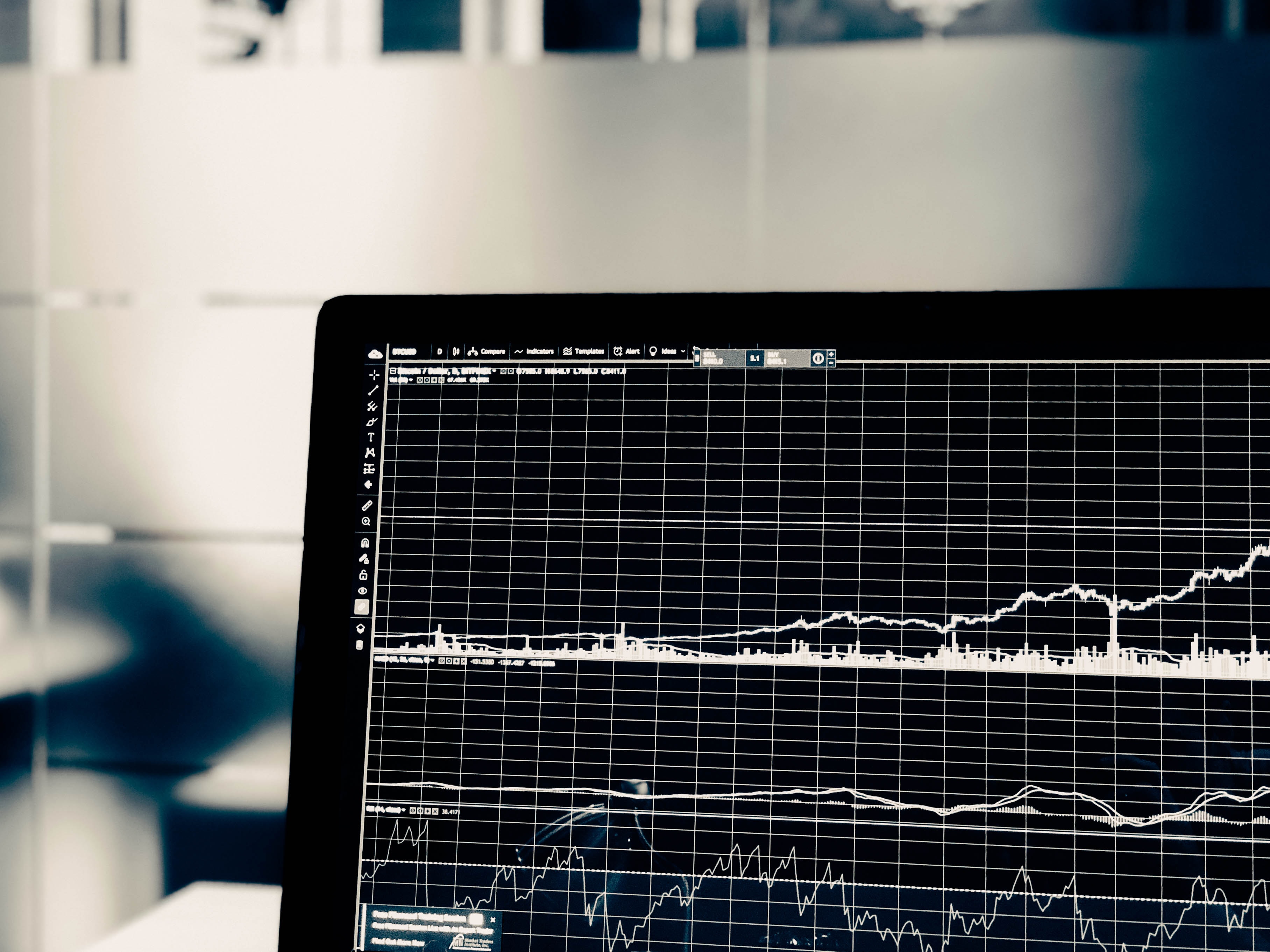
This week, I’ve been going over ecological studies in epidemiology. In my research area, ecological studies are rarely done – the prominent study designs are the usual suspects: retrospective, prospective, and case-control. Ecological studies are kind of just talked about in epi courses right before introducing the more common designs and then forgotten. At least that’s my experience. Anyone else thinks the ecological study is seldom talked about in courses? Especially in clinical epidemiology?
My hunch is it has something to do with a well-known bias coined the “ecological fallacy” that probably undermines the study design’s value far more than it should be. The infamous ecological fallacy occurs when one wrongly infers a group-level relationship also exists at the individual-level. So, while considering ecological study designs, researchers will already be thinking about the ecological fallacy! I mean a bias with fallacy in it? It will make anyone run in the other direction! I prefer aggregation bias over ecological fallacy.
Oddly enough, as I learned about this design, it was surprising that many so-called retrospective or cross-sectional studies have some ecological-ness to it. I will briefly go over this design and show how some its features may be more common than I originally thought.
Why ecological?
Intuitively, “ecological” is related to “ecology”, which is the science of the relationship between organisms and their environment. An “eco-system” is a community of living organisms and non-living organisms. When I think about ecology and eco-systems, I immediately imagine a collection of people and things. Large groups. Clusters. Using this line of reasoning, it then makes sense that ecological studies involve examining relationships at the group-level, community-level, or some broad aggregate level instead of something more granular like the individual-level.
The nuts and bolts – ecological measures
There are three common types of measures used in ecological studies and these can be considered the ecological “variables” if one is used to statistical jargon:
1. Aggregate measures: summarizes a characteristic of individuals within a group as an average or proportion. Think average fat-intake for a group of people or the prevalence of smoking in a city. Or average chocolate consumption for a country. Aggregate measures may also be measured at the individual level if we have the data.
2. Environmental measures: physical characteristics of the geography a group may be exposed to. For instance, air pollution intensity in urban areas or hours of sunlight for people living in the Northern Hemisphere. Think of an environmental measure that everyone in an area is subjected to. We can’t measure it in a person, but we can measure it for an area.
3. Global measures: These measures represent characteristics of a group that cannot or is very difficult to examine at the individual-level. For example, a type of political or health care system, a type of prevention program in a region, or exposure to a specific law or policy. These are all measures that do not necessarily have analogs at the individual level that we can realistically measure. On the other hand, aggregate measures are also global measures in a way BUT they can also be measured at the individual-level if one has the data. Global measures cannot (for the most part).
An example
A traditional ecological study compares an ecological measure of exposure (see #1, #2, or #3 above) to another ecological measure (#1, #2 or #3 above) of disease or any other health outcome. As a simple example, say we knew how many Nobel prize winners came from each of the G20 countries. Twenty countries mean we have twenty per-capita Nobel laureate rates. Now suppose we also have the per-capita chocolate consumption rate from these same countries. To easily examine an ecological association, we would plot the chocolate consumption rate of each country (ecological exposure) on the x-axis and the corresponding Nobel laurate rate on the y-axis (ecological outcome). Something like this:
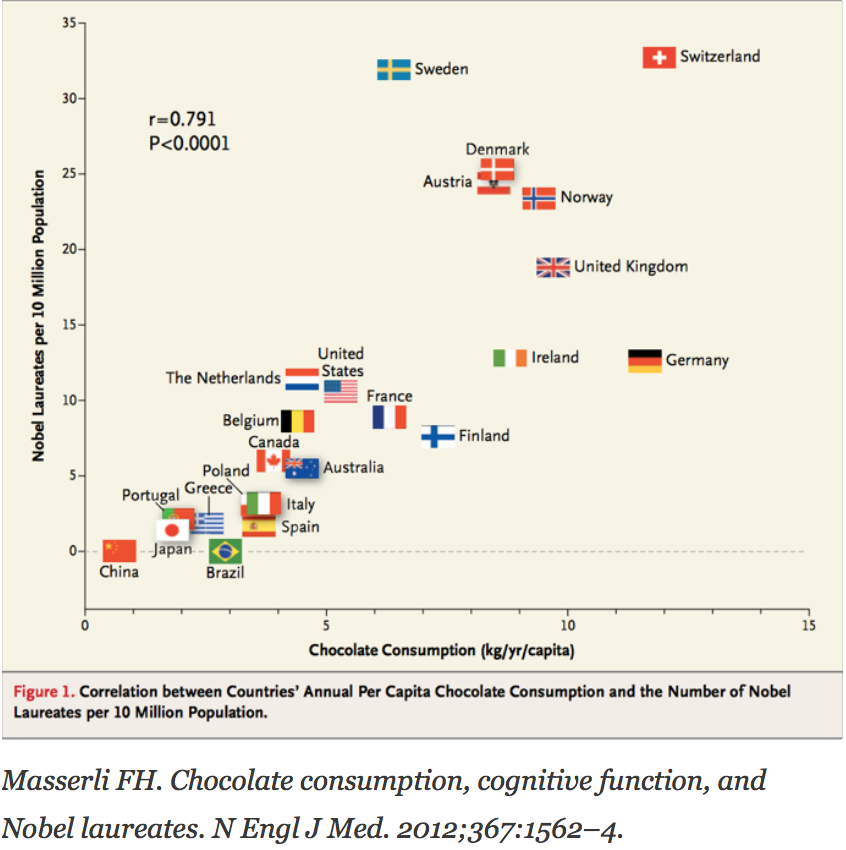
What do we observe from the plot? Assuming no information bias and confounding is present, one would probably describe this relationship as positive – as a country’s chocolate consumption rate increases, a country’s number of Nobel prize winners also increases. Pretty straight-forward. A country’s chocolate consumption seems to be related to how many Nobel prize winners they produce.
Enter: Ecological Fallacy/aggregation bias
Although there is nothing factually wrong with the above observation, people run into trouble when they start saying things like “consuming more chocolate will increase the likelihood of someone becoming a Nobel prize winner”, or “the more chocolate I eat, the more likely I will be a Nobel prize winner”! This is called a cross-level inference, where we observe an association at the group-level only and subsequently infer it automatically exists at the individual-level. Why is this fallacious or bias-ridden? The simple answer is we just don’t have enough data to parse out the real differences.
In the above example, the chocolate consumption rate for each country is actually serving as a proxy for the chocolate consumption of each individual in the country. What if I told you that we had access to each person’s life-time chocolate consumption, and it was noticed that all Nobel prize winners actually hated chocolate? It would reverse the apparent positive association. Relying on aggregate data masked the actual underlying individual data that made up the aggregate measure, which told a different story. This was obviously an extreme example, and the point was to illustrate that associations may exist at the population level. But, we shouldn’t take these associations as definitive, and at the same time, we shouldn’t just dismiss them because we were told the data is from a weak design. We should look into it deeper and ask how such an association would exist? Ecological studies provide a quick and easy starting point to generate hypotheses. Remember a single study showing a relationship, no matter what design it is, (yes, even an RCT) isn’t sufficient to prove a causal relationship. It’s a process. A long process.
A contemporary example of ecological fallacy: COVID-19 and mask usage
The unfortunate COVID-19 pandemic is providing many real-world teaching examples of common biases that arise in health research that may be misconstrued as evidence for effectiveness. For instance, many smart people are noticing that countries (i.e., groups of people) where mask use is prevalent (e.g., South Korea, Singapore, Hong Kong) appear to be doing better in controlling COVID-19 than countries where mask use is not that popular. Seems intuitive. However, ignoring strong confounding issues related to this observation (the Chinese where masks but for some reason they are omitted when making this argument, gee, I wonder why?!!), this is essentially an example of a purported group-level association (if we assume it is present) that we are inferring also exists at the individual-level. Just because the South Koreans wear masks and have thankfully turned the corner in the pandemic, then if I start wearing a mask it will stop me from getting the virus! The “to use mask or not to use mask” issue is obviously more complex than simply a bias and I will leave it to the much more knowledgeable researchers to explain the actual science behind it. For example, does it work as a public intervention? Can it be worn properly by the masses? What about shortages that invariably affect health care workers who are at more risk? I came across this news article that may serve as a good primer on some big issues around this controversial topic.
In sum, based on population-level data that masks seem to work (we don’t even know this yet), it would be an ecological fallacy to suggest it will also work at the individual-level. If we had more data on who exactly wears a mask and who does not, adjust for other factors (testing strategies, social distancing, extent of quarantining for each country), and take into account other potential costs like shortages for health care workers who need them the most then we may very well come to a different conclusion.
So when can we use ecological studies as evidence?
Ecological studies are low on the hierarchy when it comes to the strength of evidence for reasons discussed above, but sometimes it is actually is easier and makes more sense to conduct an ecological study than an individual-level study. It depends on how we want to use the results. If the implication of the study is solely to impact the population-level then ecological study designs are quite appropriate to use as reliable evidence. If, on the other hand, an ecologic design is cited as evidence to change a factor at the individual-level (see chocolate example above).…it may not be a good idea. For instance, an ecological study in 2016 established that a state’s gun ownership rates were directly correlated with its suicide rates. Now, if they had concluded that guns increase the risk of suicide and left it at that, many people would raise their arms up and yell “fallacy”! This is what they actually concluded and recommended:
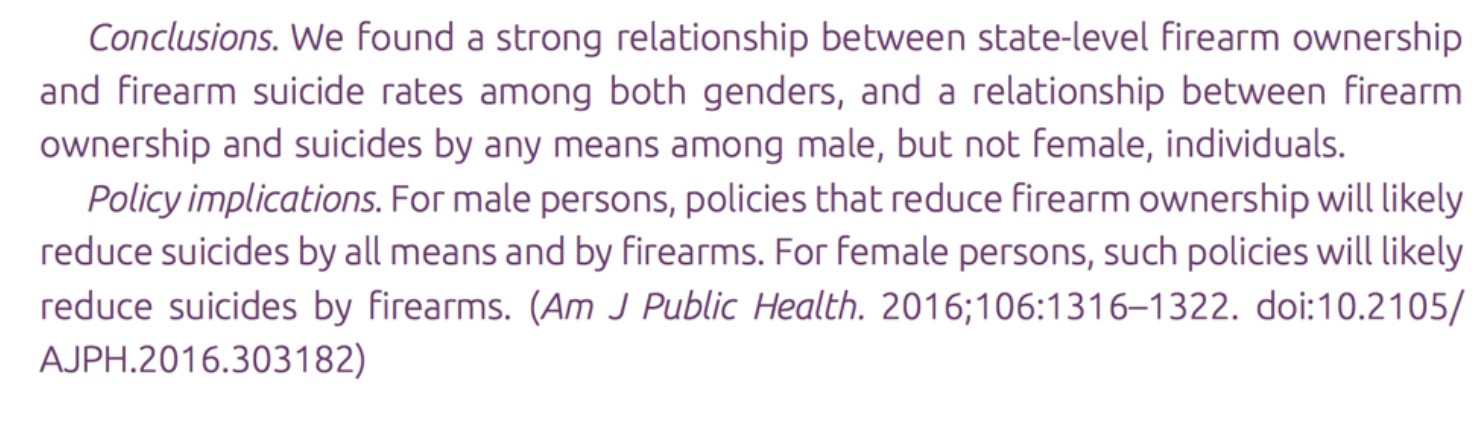
The authors’ implications of the results are at the population-level (a policy change to reduce firearms). There is nothing wrong with that. They established a relationship at the ecologic/population-level and they also want to intervene at the population-level. Despite a weak design, an ecological study in this scenario, where the point of intervention was also at the population-level, is probably more accurate and appropriate. Both population and individual-level factors contribute to the causal web between guns and suicides, and they both are valid, particularly if one is more amenable to change or implementation.
Re-visiting the COVID-19 and mask example, let’s say a well-conducted ecological study (-ies) taking into account other public health interventions such as testing strategies and physical distancing measures as well as the type of masks, etc., found that indeed population-level mask usage adds value in controlling the COVID-19 infection spread. This study would provide compelling evidence to implement some sort of public policy that encourages the use of masks for the masses. However, it would also mean that everyone in the population would have access to these masks (without shortages to front-line health care workers) and introduced with educational campaigns on how to use masks, what types are the most appropriate, and so on to really replicate the observed population-level benefits of masks. Key-point: the implication and use of evidence was at the population-level.
Individual-level studies with ecological-ness
Ecological studies are a rarity in my area, but I was pleasantly surprised to find that a lot of individual-level studies actually integrate ecologic measures. For instance, many retrospective before-after studies examining a law change or program implementation use an ecologic exposure (“global measure”) on individual risk of an outcome (e.g., cannabis use, injuries, emergency visits). The law change or program is really the ecologic exposure of interest. Some other examples of global measures often used in epidemiologic research: median family income of neighborhoods, rural vs. urban, and aggregate socioeconomic indicators. Sometimes we use these ecologic measures as a proxy because individual-level measures are not available. Or sometimes we may feel that the ecologic measure more accurately depicts the phenomena we are interested in. Nonetheless, ecological measures are more common than I thought
Conclusion
Ecological studies get a bad rep because people automatically equate a fallacy to it. However, we just have to be careful with our language and how we make our ultimate inference and conclusions to use them appropriately. Many individual-level studies also use ecologic measures, and we need to be just as careful when making inferences with these studies. When applied to make inferences at the proper level (e.g., population-level), ecological studies give valuable insights to a health problem. They may also provide impetus to pursue individual-level hypotheses in more detail.